Artificial Intelligence in Agriculture in India
Artificial intelligence in agriculture and allied activities is having its moment in the sun. At a time when the whole world is disrupted by COVID-19 and social distancing is the norm, electronic mandis, for example, could offer an excellent way for transactions between farmers and customers in the post-COVID world.
The e-mandi concept has previously been tested in different parts of India, and would add transparency and efficiency to the whole marketplace. AI in Agri-tech could bring the kisan back to his glory days as the nation rebuilds its economy after the current crisis.
Indian government keen on AI and Big Data in rural farming
While addressing the third ‘India Agricultural Outlook Forum 2019 with the theme “Universal Basic Income for Farmers,” Agriculture Secretary Sanjay Agarwal had stated that AI and Big Data will play major roles in the agriculture sector in coming years since data is “key to targeted development”.
The Indian government is collating volumes of data on farmers through the registration process for national-level schemes. Land holding details are also being digitised. The government is studying information on farm insurance, soil health card and Kisan Credit Card (KCC) to understand the status of farmers and their crops. Pradhan Mantri Fasal Bima Yojana (PMFBY) is using remote sensing imagery, AI and modeling tools to reduce claim settlement times. PMFBY covers nearly 20 million farmers.
What is artificial intelligence in agriculture?
Agriculture may be the most ancient of occupations, yet its relevance has only become bigger with the imminent threat of food insecurity. Technology powered by Artificial intelligence is ensuring the sustainability of quality food production for the coming decades. AI solutions are being used to diagnose pests, predict the best time to sow and gauge prices for produce. Drones, hydroponics, artificial lights and AI-powered cameras are protecting crops from wild animals.
The agriculture industry is turning to AI for solving the double trouble of the food crisis and food wastage in the wake of locust swarms, climate change, droughts and floods. Just as AI solutions have seeped into every aspect of our lives — music discovery and restaurant recommendations to banking and fitness, so also with agriculture. In fact, the contribution of artificial intelligence in agriculture is only set to increase with agri-tech startups cropping up (pun intended) in Bengaluru, Hyderabad and New Delhi.
How can AI be used in agriculture?
Cognitive computing in particular, is all set to become the most disruptive technology in agriculture services as it can understand, learn, and respond to different situations (based on learning) to increase efficiency. Some of them can be services for the producers. For example, the chatbot that pops up when you visit a banking website could be incorporated into a kisan app. The land owners can have a virtual conversation on the platform and have their basic queries answered instantly. They can also keep tabs on the latest innovations they ought to know about.
https://youtu.be/hhoLSI4bW_4
6 Main Areas where AI can Benefit Agriculture
1) Growth driven by IoT
Huge volumes of data are generated every day in both structured and unstructured format via IoT (internet of things). These relate to data on historical weather pattern, soil reports, new research, rainfall, pest infestation, images from drones and cameras and so on. Cognitive IOT solutions can sense all this data and provide strong insights to improve yield.
2) Soil testing
Two technologies that stand for intelligent data fusion are Proximity Sensing and Remote Sensing. One use case of this high-resolution data is Soil Testing. While remote sensing requires sensors to be built into airborne or satellite systems, proximity sensing requires sensors in contact with soil or at a very close range. This helps in soil characterization based on the soil below the surface in a particular place.
3) Image-based insight generation
Drone-based images can help in in-depth field analysis, crop monitoring, scanning of fields and so on. They can be combined with computer vision technology and IOT to ensure rapid actions by farmers. These feeds can generate real time weather alerts for farmers.
4) Detecting crop diseases
Images of various crops are captured using Computer Vision Technology under white/UV-A light. Farmers can then arrange the produce into separate stacks before sending it to the market. Pre-processing of images ensures the leaf images are segmented into areas for further diagnosis. Such a technique would identify pests more distinctly.
5) Optimal mixture of agri products
Based on multiple parameters like soil condition, weather outlook, type of seeds, infestation around a certain area, cognitive computing makes recommendations to farmers on the simplest choice of crops and seeds. The advice is further personalized basis on the farm’s requirement, local conditions, and past successes. External factors like marketplace trends, prices or consumer needs can also be factored in through artificial intelligence.
6) Monitoring crop health
Remote sensing techniques alongside hyper spectral imaging and 3D laser scanning are essential to create crop metrics across thousands of acres. It could usher in a revolutionary change in terms of how croplands are monitored by farmers in terms of time and energy. This technology will monitor crops along their entire life-cycle and generate reports for detecting anomalies, if any.
3 successful public-private partnerships for digital farming
Here’s how digital farming in India has helped increase crop yield by as much as 30%!
1. AI-sowing app by Microsoft
Microsoft and a local non-profit, non-governmental agricultural research organization, International Crops Research Institute for the Semi-Arid Tropics (ICRISAT), collaboratively developed an AI-sowing app. The app is powered by Microsoft Cortana Intelligence Suite and Power Business Intelligence.
The Cortana Intelligence Suite includes technology that helps to increase the value of data by converting it into readily actionable forms. Using this technology, the app is able to use weather models and data on local crop yield and rainfall to more accurately predict and advise local farmers on when they should plant their seeds.
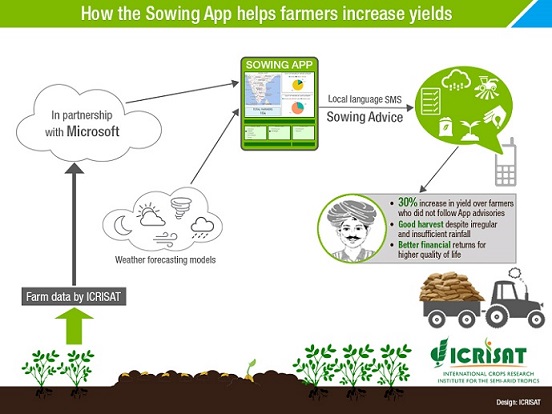